Team member:
Xie Wenjun(Experimentalist)
Team profile:
Xie Wenjun is a member of the VCC-XR Team, his main research direction is computer animation (including human pose estimation, motion data analysis, human motion synthesis). In recent years, he has led and participated in projects involving VR/AR interactive program development, computer game design, virtual people and natural interaction, information visualization, etc.
Recent work:
Audio-Driven Collaborative Generation of Virtual Character Animation
Considerable research has been conducted in the areas of audio-driven virtual character gestures and facial animation with some degree of success. We propose a deep-learning-based audio-to-animation-and-blendshape (Audio2AB) network that generates gesture animations and ARKit’s 52 facial expression parameter blendshape weights based on audio, audio-corresponding text, emotion labels, and semantic relevance labels to generate parametric data for full-body animations. By using audio, audio-corresponding text, and emotional and semantic relevance labels as input, the trained Audio2AB network could generate gesture animation data containing blendshape weights. The experimental results showed that the proposed method could generate significant gestures and facial animations.
Research achievements:
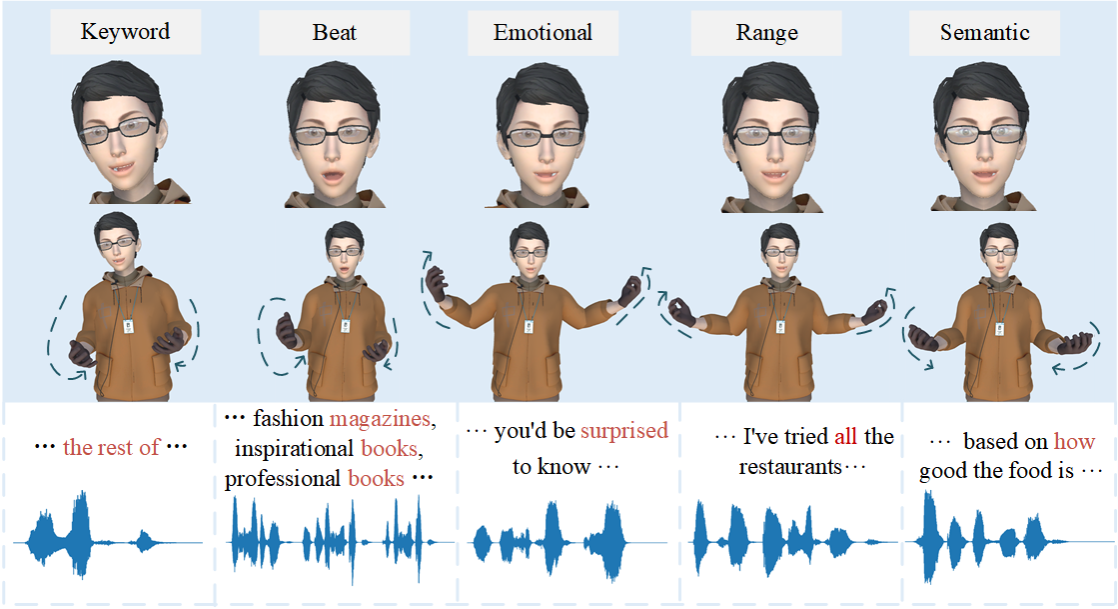
Multimodal Fullbody & Hand motion Datasets
Human motion dataset is an important foundation for researches such as motion data denoising, motion editing, motion synthesis, etc.
A full body motion dataset named HFUT-MMD is captured, which contains 6 971 568 frames of fullbody common motion data in 6 types from 12 actors/actresses, 227 160 frames of fullbody high noise motion data, and 967 550 frames of hand motion data. The experimental results on the HFUT-MMD dataset using the existing algorithm show that the low precision motion data can be optimized to obtain the motion data similar to the accurate motion data, which corroborates the consistency between the modal data.
Download link: https://gitee.com/plusseven/HFUT-MMD
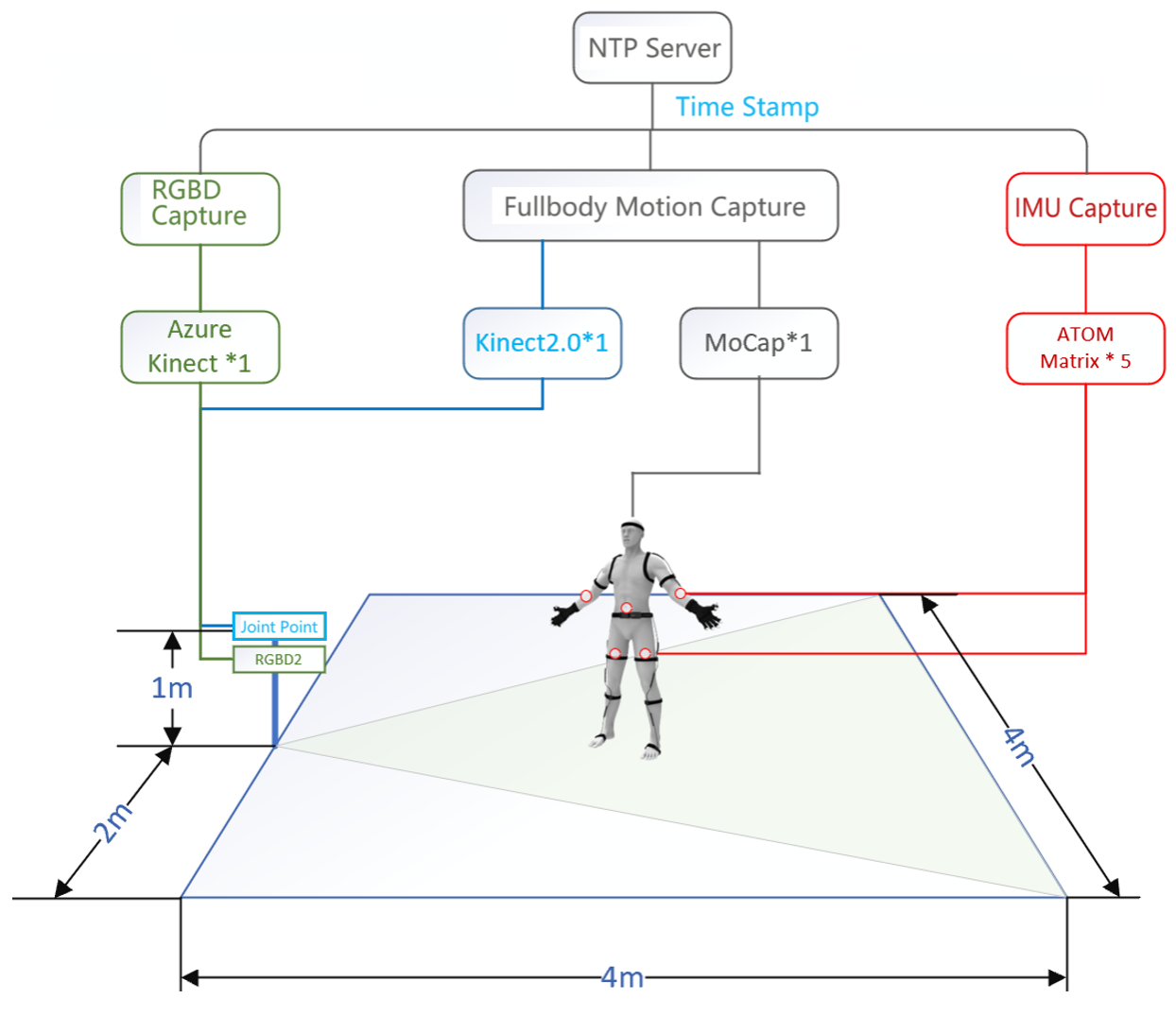
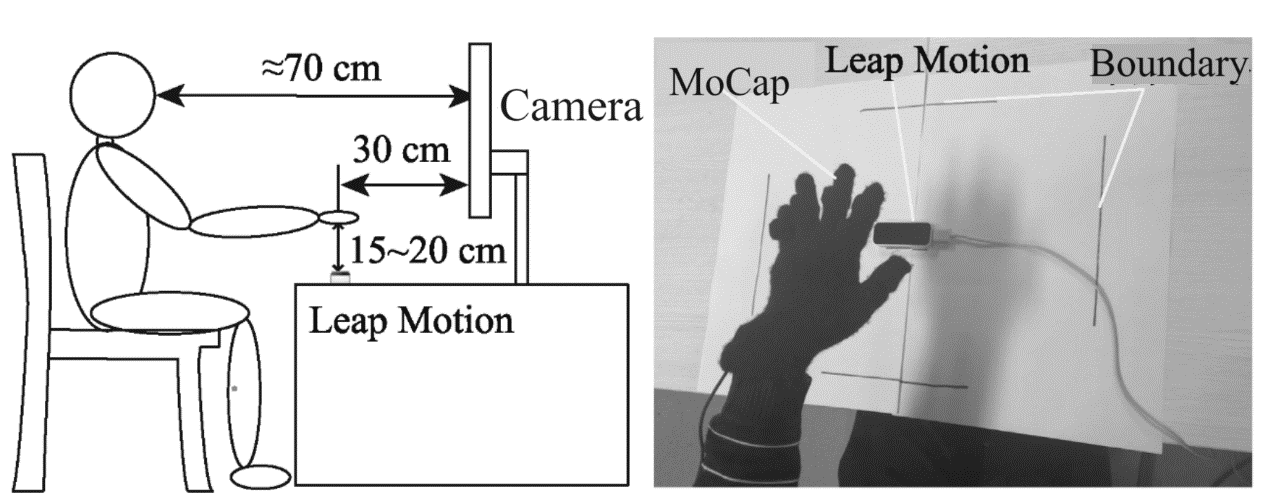
Instructed Student Work
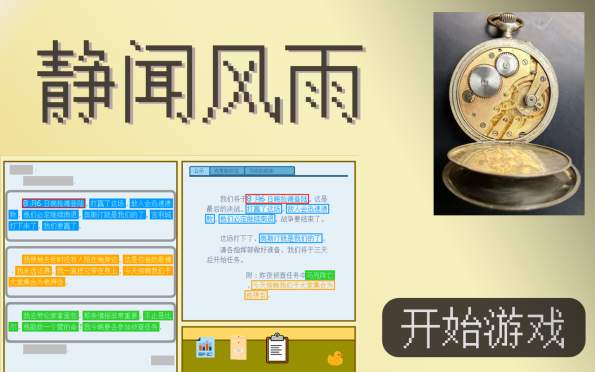
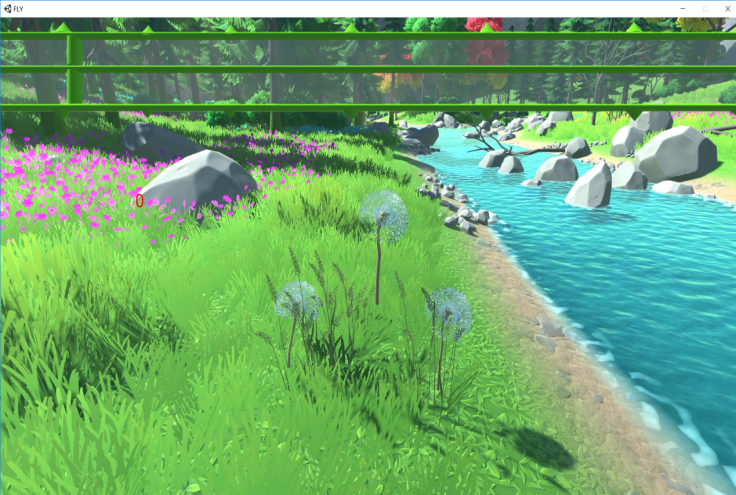
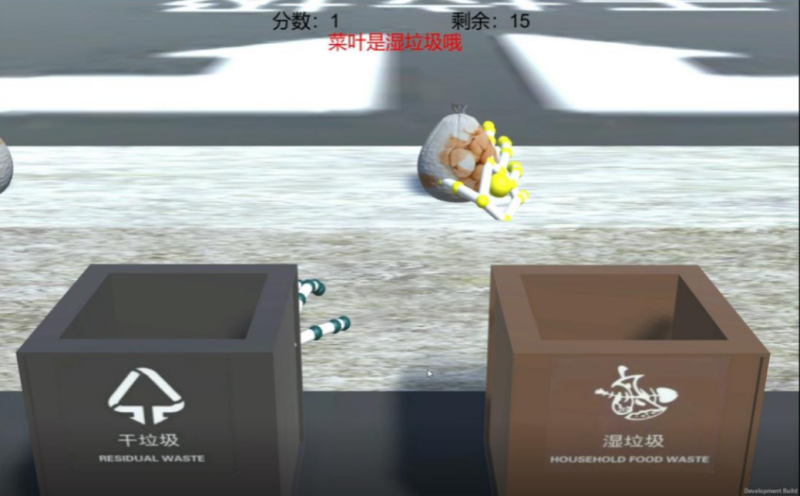
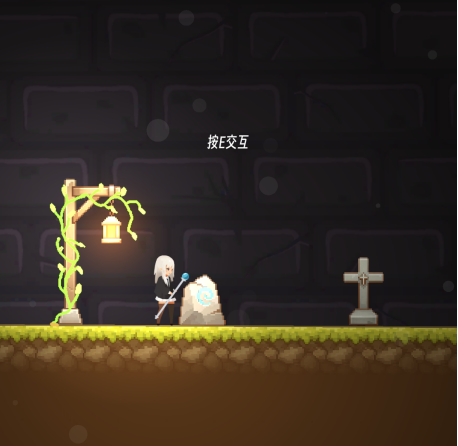